Dr.-Ing. Dipl.-Wirt.-Ing. Götz Andreas Kemmner
Production will always remain a process that cannot be planned in detail. However, this does not mean that throughput times, inventories, adherence to delivery dates and capacity utilization in production cannot be controlled.
The task of production planning and control is to regulate the flow of materials and orders through production as optimally as possible. The targets are clear to everyone: short throughput times, high adherence to delivery dates, low work in progress and good utilization of production capacities. Anyone who has ever worked in production planning and control knows the problem:
You are happy about a higher capacity utilization due to the order situation and then the work in progress increases, the adherence to delivery dates decreases and the lead time increases. Particularly in the case of one-off and small series production, even less is produced at the end of assembly, as there are always parts missing to complete the order. In some companies, there is a feeling that these targets elude all planning and control.
The term “scheduling dilemma” is used, although it is actually more of a “quadrolemma”. Imagine you have to push three beach balls under water in the bathtub and at the same time make sure that the water level doesn’t rise… So that the task doesn’t become too easy, someone constantly turns on the tap or drains water and blows air into the water balls or lets some escape. An impossible task, you might say, but this is precisely the task that many companies set their production controllers every day with a cold smile.
Some innovative companies have tried and continue to try to help their production controllers by providing them with detailed planning software and control centers. Now, calculations are sometimes carried out for hours, even nights, in order to optimize the scheduling of production orders and work processes to the minute. However, the boundary conditions have changed faster than the results can be presented. The systems run after a reality that always develops differently than the calculation expects. A precise miss is also a miss!
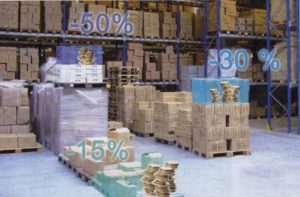
“Production is a process that cannot be planned in detail”, this old Wiendahl statement is just as immovable as Heisenberg’s uncertainty principle in quantum physics, but while the latter is generally accepted – although none of us understood it so precisely at school – numerous software products and companies using them live on the hope of being able to overcome Wiendahl’s uncertainty principle in production. You should be aware that this is only possible with specific, statistical approaches!
Both supposedly fundamentally different theorems are based on a common mechanism. Many physical quantities have a “fuzzy”, i.e. statistical, relationship. The relationship between lead time, adherence to delivery dates, work in progress and capacity utilization in a production facility with numerous production orders, operations and capacity locations is also statistical in nature. These variables can therefore only be optimized using statistical methods. So if you, as a production controller, are criticized for poorly controlled production, recommend that the person you are talking to first of all take a look at Heisenberg’s uncertainty principle.
You will probably not be able to get rid of your conversation partner permanently this way, especially not if he follows your recommendation and actually familiarizes himself with the subject matter in more detail. However, you have gained time to understand the context in more detail before he returns. The fact that lead times, on-time delivery, work in progress and capacity utilization are “only” statistically linked only explains why you are not performing well using detailed planning software and control station functionality, but it also shows that you could perform well with the right tool and the right method!
But you have gained time and we want to use it; not by digging deep into the statistical correlations (universities employ enough professors for that), but by jumping straight to the end of the considerations and dealing with the practical findings of the statistical considerations.
The graph in Figure 2 shows a production characteristic curve. Technicians may be reminded of engine characteristic curves and this analogy is not that far off. Such a production characteristic curve can be created for an individual capacity point (workstation, machine), for a group of capacities, for a production department or even for the entire production.
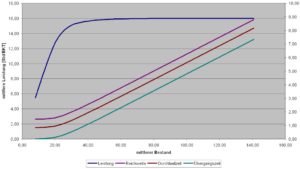
The left-hand curve of the characteristic curve graph shows the power above the average stock. The average stock is the worklist in hours that is on average, over a longer observation period, in front of the capacity point under consideration. The capacity describes how many hours of work content are processed by the capacity center per operating calendar day. It can be clearly seen that the output initially increases in proportion to the average stock. The performance curve then becomes increasingly flat. If you “shovel” so many orders in front of the machine group in this example that the average stock increases from 300 hours to 600 hours, you gain an incredible 2 hours of output per operating calendar day. Unfortunately, not only has a mountain of stock been accumulated, but also, as the three parallel curves below show, the lead time has been extended from approx. 1.5 days to approx. 3.5 days (as can be seen on the right-hand vertical axis in the diagram). Do such connections sound familiar to you?
Adherence to deadlines, which is particularly difficult to grasp statistically and is therefore shown as a dashed line in the graph, was poor at 300 hours of order backlog and falls back to a similarly poor value at 600 hours. At 300h order backlog it was bad because a whole series of orders were completed too early; at 600h it is bad because most orders are now completed too late.
Before your interlocutor returns from studying Heisenberg’s uncertainty principle, we need to make practical use of the production curve under consideration: Every production, every capacity group, every capacity point has such a production characteristic curve. The basic curve shapes are always the same; they differ in their numerical values and their curvature. Unless you intervene with organizational or technical measures, the curves remain constant in the medium term, so you can push, shove, pull or chase deadlines as much as you like. Throughput time, inventory, adherence to delivery dates and performance (capacity utilization) are in the statistical relationships described by the curves.
This leads to the first key insight: if you want to manage production sensibly, you must not rush back and forth between the various target values of lead time, inventory, adherence to delivery dates and capacity utilization on a daily basis, but must position yourself correctly and strategically, depending on the current economic and competitive situation, by determining what compromise you want to make between the various target values. You mean you already do that? And what about knocking down stocks at the end of the month and, above all, the financial year, while otherwise making the best possible use of capacities?
The second key finding is that you change your logistical positioning by changing the average stock of a work system. This is your slider, so to speak, but you can only move it to a certain degree of precision. You can only move this controller precisely at the first workstations where a production order is processed. The more work stations a production order passes through, the more potential disruptions affect it and the more uncertain it becomes as to when it will be available for processing at a work system and contribute to the inventory at this work system. You do not move the sliders deeper in the production process directly, but – once again – only via statistical mechanisms.
By changing the average stock, the other logistical variables shift along the characteristic curves, as shown in the example. If you are not satisfied with the result because you want to achieve shorter throughput times or better performance with the targeted stock, for example, you need to intervene with technical and organizational measures, for example by
- reduce time-related peak loads,
- Reduce minimum transition periods,
- Harmonize order times,
- Reduce order times,
- increase capacity flexibility or
- enable overlapped production
- enable parallel production.
As a result – and this is the third important finding – they cause a change in the statistical relationships and thus shift the characteristic curves. You have reached your goal when you have succeeded in shifting the characteristic curves so that they run through the desired operating point. If you do not succeed in doing this, you will not reach your operating point.
Now you already know a lot about how to stand up to your critics. Your boss should be impressed by your new knowledge. However, he will probably now ask you why you are not putting your findings into practice. But you don’t have to owe an answer here either:
Of course, you could dig through several kilos of statistical formulas and write an evaluation program that reads several hundred thousand work process dates and master data from the PPS system and determines the statistical characteristics from this. In principle, it would not be too much for you to write a scheduling algorithm that makes it possible to control the load on the production capacities “further back” in production via the order scheduling at the first production capacities. All you need is two or three of your colleagues and perhaps a few months’ time. If he doesn’t understand this, you can tell him in simpler words: Give me the right tools and stable specifications and I will control the production correctly.
Keywords:
Production planning, production control, lead time, adherence to delivery dates, work in progress, capacity utilization
A Guide for Challenged Production Planners
Manufacturing will always be a process that cannot be planned in detail. That doesn’t mean the likes of throughput time, inventory, adherence to schedule, and utilization of capacity in manufacturing cannot be controlled. This article explains how to shift static relationships by correct strategic positioning a variation of the mean volume of work on an operating system so that they concur with the operating point aimed at, and where you can find the tools you need to efficiently control the manufactoring process.
Keywords.
production planning, throughput time, inventory, adherence to schedule, and utilization of capacity in manufacturing
Dr.-Ing. Dipl.-Wirt.-Ing. Götz Andreas Kemmner is managing partner of the management consultancy Abels & Kemmner GmbH, Herzogenrath/Aachen.