How big data becomes smart data:
Scheduling 4.0 for the factory 4.0
Gerrit Sames, Andreas Kemmner
Never before have we generated so much data in companies – and unfortunately done so little with it. But how do you turn big data into smart data? This topic will be taken up using one example: disposition. Mechanisms for Disposition 4.0 already exist, but they are only being used by a few performance leaders. The main challenge and therefore the decisive key to MRP 4.0 lies in the intelligent evaluation of the enormous amounts of data that are available in today’s ERP systems due to the increasing digitalization of processes and are constantly growing.
Let’s take a look at the initial situation: With the increasing digitalization of processes, companies are generating more and more data. This applies equally to data from production processes and data from administrative processes. Unfortunately, the systematic collection and analysis of data is still underdeveloped, and little or no conclusions are drawn from the data. What is important here is that the data analytics must fit the questions very precisely and therefore be designed very specifically, as we will see in more detail later. Intensive work is being carried out on suitable mathematical methods and algorithms to filter information from the data volumes, and the first practical solutions already exist.
Scheduling is the heart of every company
It is easy to understand why the enormous amounts of data and their intelligent evaluation are so crucial for MRP 4.0 when you realize that MRP is the heart of every company, pumping the entire material flow through the supply chain and value chain. This is why the quality of scheduling is of crucial importance for the profitability of a value chain. The quality of scheduling depends on the scheduling parameters, as they play a decisive role in determining how stocks, delivery readiness, ranges, capacity utilization and throughput times in procurement, production and distribution develop and how economically the entire value chain works.
Many companies do not achieve the effects they had hoped for from their ERP system
In today’s Factory 3.0, there is already a lack of effective scheduling: many companies are finding that, despite the use of ERP systems, the desired reduction in inventory, for example, is not materializing. Planned delivery readiness levels were also not achieved. Our companies are well aware of the main reasons why ERP targets are not met: Maintenance of MRP parameters often does not take place or only at too great intervals. First of all, this is due to the excessive amount of manual maintenance and therefore time required for data maintenance. However, even in companies where maintenance is carried out, the quality of the scheduling parameter settings is usually poor, because on the one hand far too few parameters are considered, and on the other hand these parameters are usually still set to the best of the responsible scheduler’s knowledge.
No great visions are needed to recognize that the challenges will continue to grow. The time available to dispatchers for data maintenance will inevitably become less and less. Demographic trends alone will lead to an increasing shortage of the necessary personnel. But what is probably even more decisive is that users’ understanding of the effects of dispensing parameters is very limited and even proven experts can no longer reliably understand their complex interaction. Ultimately, ERP systems generally do not provide suitable tools for optimizing scheduling parameters.
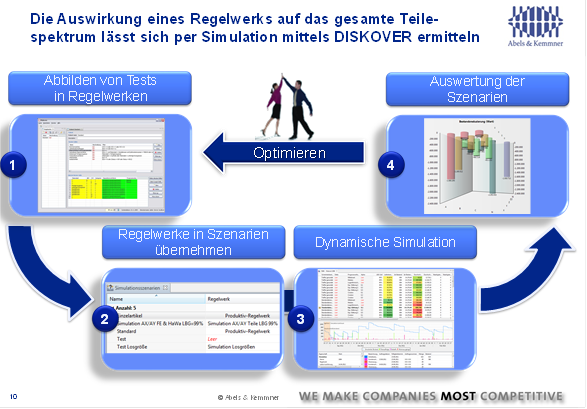
Conventional maintenance of scheduling parameters is too time-consuming
Imagine you were responsible for 1000 items and wanted to concentrate on maintaining the 10 most important scheduling parameters. You want to view these four times a year, i.e. once every three months. It is certainly no exaggeration to assume a time expenditure of 60 seconds per MRP parameter, because each material number has to be called up, the masks with the desired parameters have to be loaded, and the correct setting has to be thought about or looked up. If you add everything up, you arrive at a maintenance requirement of 666 hours per year. This corresponds to approx. 40 percent of your annual work capacity.
Every practitioner is aware of this effect, but most companies do little to counteract it: Every user weighs issues differently, handles them differently and only has an overview of part of what is happening. Economically optimized scheduling results cannot be achieved by gut feeling, because the interaction of the various scheduling settings is extremely complex. Ultimately, it is about statistical effects and statistical correlations between parameter settings and economic results. Even if you only assume 10 parameters that are important for the performance of an item, as in our example of the time required for data maintenance, no one can assess the logistical interaction of these parameter settings and therefore their economic impact. In powerful ERP systems, however, far more scheduling parameters can be set for each material number. In the SAP system, for example, up to 130 parameters can be defined for each material; this does not even include settings for historical values, quotations, scheduling agreements and contracts. Of course, nobody needs that many settings for an article at the same time, but in practice there are always far more than 10. At first glance, it seems difficult to achieve correctly set disposition parameters under these circumstances.
Big Company Data Analytics opens up broad opportunities for scheduling 4.0
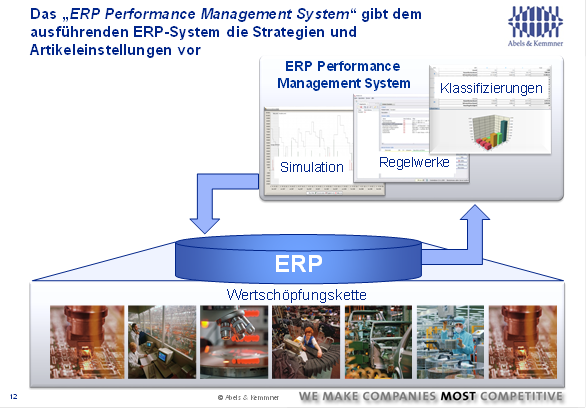
However, the DISKOVER tool is the first Factory 4.0 solution on the market that is able to use the extensive data in the ERP system to determine optimized parameter settings and continuously readjust certain scheduling parameters (see Fig. 1). DISKOVER is already being used by well-known companies such as Hansaflex AG, Trost SE and the STO Group. The results achieved with the use of DISKOVER are remarkable. For example, an international production company was able to achieve an inventory reduction of over 40% by optimizing the scheduling parameters and setting an availability level or delivery readiness level of 95%.
At the heart of the analysis are simulations that are used to check how a certain combination of scheduling parameter settings affects the profitability of the scheduling results. The DISKOVER system crashes the scheduling in the computer, so to speak, before the parameter settings are implemented in practice.
The simulation process does not replace the expert who can interpret the simulation results and draw conclusions from them. However, optimization processes are drastically accelerated, risks are significantly reduced and far better quality results are achieved. On the one hand, the simulation results can be mapped in scheduling rules and, on the other hand, particularly dynamic parameter settings, such as safety stocks or forecast values, can be directly readjusted using simulation processes.
It is also very interesting that it is possible to check directly for each individual article and each material whether the required delivery readiness levels can actually be met in practice and which approximate target stock levels can be achieved. The following section takes a closer look at how such a simulation approach works in practice. The basic data analysis and simulation process can be divided into five steps:
- Appropriate methods can be used to calculate stock trends, delivery readiness levels and ranges of coverage from stock inflows and outflows.
- Simulations with variations in dispatching parameters and scheduling strategies can be used to calculate target ranges of coverage or the number of vehicles to be dispatched. The degree of readiness for delivery can be determined.
- With which settings, under which boundary conditions, optimized stock heights, ranges of coverage resp. The degree to which delivery readiness levels are achieved is mapped in decision tables and regulations.
- The rule-based setting parameters are fed back into the ERP system; manual maintenance of dispensing parameters is therefore no longer necessary.
- The results are optimized and fed back into the ERP system automatically on a daily basis or at selectable times.
In order to simulate the effects of alternative MRP settings for different article groups, MRP parameters to be tested are set in the DISKOVER system.
or entire sets of rules are transferred to scenarios and fed into the simulation process. The results can be viewed directly in DISKOVER as an overall result for all articles as well as for each individual article in order to obtain information for optimization approaches. In this way, different alternative courses of action can be played through and compared with each other.
The result of the data analyses is not only information on the correct parameter settings in the ERP system, but also strategic insights and organizational rules, which we do not want to deal with here, although they can be of great importance for corporate strategy. Of direct importance for scheduling 4.0 is how to deal with the technical findings with regard to scheduling rules in order to be able to apply them effectively and efficiently in practice.
A central task of Dispo 4.0 systems: ERP performance management
Rather, the consistent implementation of MRP 4.0 requires a strategically oriented tool that specifies the current MRP parameter settings to the ERP system and thus optimizes logistics performance – one could call it an “ERP performance management system” (see Fig. 2). Such an ERP performance management system readjusts the parameter settings in the ERP system. It must
- transfer a wide range of basic data from the ERP system;
- carry out numerous item classifications and key figure determinations;
- rules and decision tables;
- have extensive simulation functions;
- return the setting specifications to the ERP system.
Conclusion
Industry 4.0 will move into our companies. Big data and big data analytics will become an important component of this. Very specific algorithms are required to turn big data into smart data. These are available for scheduling 4.0, for example with DISKOVER, and are already in use in many companies.