Companies often have several thousand or tens of thousands of articles to plan. Depending on the ERP system, there are 20 – 100 MRP parameters per article that have to be set and regularly checked for correctness and consistency. Doing this manually is a Sisyphean task. Nevertheless, the parameters have to be set in order to keep the delivery readiness high and not let the inventories explode. What is the best way to do this and, above all, to do it sustainably?
When I started my professional work as a management consultant at the end of the nineties, companies were just preparing for the year 2000 and the introduction of the Euro. And in many companies these were decisive arguments to introduce a new ERP system. An essential point was the transfer of the old master data into the new system: a step that was often still performed by means of migration databases at that time. The checks of the data transferred from the old system were often carried out using rules. And I can still remember the so-called exception reports, in which master data inconsistencies were listed that had to be checked and resolved. Since ERP implementation projects in those days often lasted a year or more, there was plenty of time to run the master data reports over and over again and correct the data. And many companies managed to go live with relatively clean master data, thanks to the great effort put into the project.
But after the go-live, problems often arose and still arise. The migration database with its reports was or is suddenly no longer there to point out problems, gaps or inconsistencies in the master data. And in many ERP projects, the topic of reporting is only properly addressed at the very end or even after go-live. Or was it different in your company, and did you have all the reports ready for go-live? And all of a sudden, many departments each have to maintain a part of the master data. For specialized departments whose main task is data management, validated processes and systems should cover the fact that the data is maintained consistently, regardless of whether it concerns a material change or a new introduction.
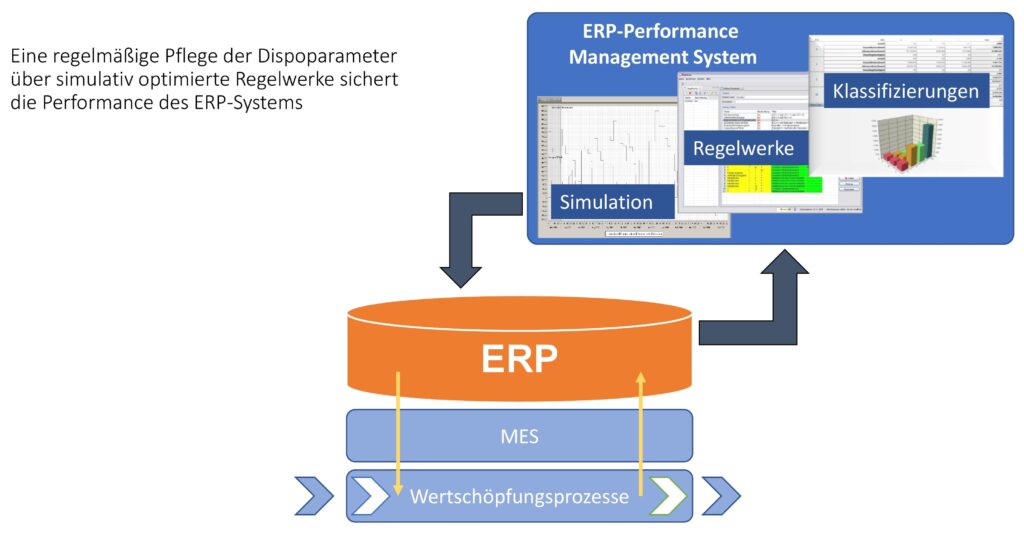
But what about in the multitude of other cases? In departments where master data management is more of a sideline activity, there is often no time or priority in day-to-day business for a regular review of master data. When new ERP systems are introduced, master data is often merely copied from the legacy system. And even when master data is maintained, it is difficult to overlook the resulting economic consequences of supposedly correctly set or inconsistent master data. As a result, errors and inconsistencies gradually creep into the master data.
Now, to be fair, it should not be claimed in this context that the master data of all companies is equally bad. In some industries – such as pharmaceuticals, chemicals or food – the master data required for production is usually of extremely high quality. You wouldn’t even want to think what would happen if there was a decimal point in the wrong place in a bill of materials there. Well trained QA/QC departments, engineering change processes and mature quality systems do the rest. Up to a certain point, you can even enforce a certain master data consistency.
But as soon as we stop talking about GMP-critical master data or as soon as the quality of certain master data fields is no longer checked or ensured via QA/QC systems or master data tools, the quality of the master data is often much less good.
Costs caused by master data problems are often underestimated
Individual master data problems do not have to lead directly to a major (production) problem or significant economic damage. And often, master data that no longer corresponds to the current reality is only perceived as an annoying inconsistency. Unfortunately, the law of large numbers also applies here: Many smaller inconsistencies can cause significant problems and costs in total.
In our consulting practice, we often make use of data from ERP systems as the basis for our analyses. One of the first questions we therefore ask our customers is: “How good do you think your master data is?” And only in the rarest of cases do our customers then dare to put their hand in the fire for their master data. And this is despite the fact that no customer doubts that this data is important.
Values that are correct in terms of system technology do not always make economic sense
Take master data in the areas of planning and scheduling, for example. Think of fields like delivery time, minimum lot size, rounding value or safety stock.
In real life, it is not enough to fill in these data fields correctly once during article creation. The sensible value is rather mostly dependent on the current economic situation or the current phase of the life cycle of a material. A minimum order quantity of 1000 pieces as well as a rounding value of the same size sound at first as correct and consistent values. But do these values still make sense if the annual demand has reduced from 10,000 pieces 3 years ago to 500 now?
It is even more difficult to achieve consistency of master data across BOM levels. Let’s take a simple example: To produce 1 piece of an end product, you also need 1 piece of a certain component. Would it then make economic sense if the lot size of the end product was 1000 and the lot size of the component was 900? Considered independently, both lot sizes can make sense, but in combination they are suboptimal because the orders/productions of the component are asynchronous from the orders/productions of the final product. And now imagine how complex such considerations become when there are multiple relationships between components and end devices.
Rules and simulations automatically ensure economically meaningful, consistent data
How can such inconsistencies and inconsistencies in the master data be counteracted? The only practical approach is to use sets of rules. Such sets of rules take master data from the ERP system and systematically check the transferred values according to certain rules that the user can define himself. Such checking rules can be simple, e.g.: “If the material belongs to material group X and the vendor is Y, the default delivery time is set to Z days”. But it is also possible to define much more complex rules. In addition, the rules can be adapted to current economic conditions without much effort, resulting in consistent, adaptive behavior in times of Corona, for example. And the use of checks on an aggregated level such as product group, material group, customer group or supplier leads to the fact that one can quickly and consistently check all instances of the corresponding group and, if necessary, change values. In addition, when a new material is created, certain master data is directly set correctly, which significantly reduces both the effort and the risk of errors in master data creation.
When checking complex relationships between master data whose interaction is not directly apparent, the use of simulation systems is also recommended. In such systems, the interaction of various scheduling parameters can be simulated on the basis of historical data. In this way, the effects of different parameter settings on the value stream behavior can be revealed and the parameter settings can be improved as a result. Within the framework of such simulations, it is also possible to check what results other master data would have led to, e.g. with regard to inventories, delivery readiness, number of orders or number of production orders. In addition, it is possible to quickly and efficiently show what the changeover from fixed parameter values (such as: “100 pieces”) to dynamic values (such as: “stock up for one month’s demand, but round off to 10 pieces”) would mean, without having to intervene in the operative ERP environment and humbly wait for the practical results or the resulting damage. Simulation can be used to quickly and cost-effectively gain fundamental insights into interrelationships between different master data fields that lead to serious economic improvements, such as inventory reductions often exceeding 20%. With this approach, the management of master data is solved in a low-effort, consistent, sustainable and yet adaptive manner. If the simulations are also carried out regularly – monthly, for example – the risk of master data management being lost in the day-to-day business is also significantly reduced. However, a well-trained user should take over the maintenance of the rules and simulations, which is why companies should not only purchase tools but also train their users.
What can be done when rulebooks or simulation are not options?
Even in cases where automated solution approaches such as rule sets or simulations are not possible or not economical, it is possible to improve the quality and consistency of the master data by simple means. Many companies calculate intelligent key figures, such as the range of the smallest possible lot size, which indicate economic inconsistencies in the master data. If, for example, the smallest quantity that you can order according to your master data settings would last for more than 2 years, the question quickly arises whether this lot size still makes economic sense.
Another pragmatic step is to regularly send your “suppliers” an overview of the master data in your system for review. The term supplier in this context can of course mean both an internal supplier (such as the production department) and an external supplier. Many suppliers also have a strong interest in ensuring that their customers have realistic delivery times interfaced in their ERP systems and order in meaningful quantities – such as full pallets or pallet layers. Even in retail environments, with typically many suppliers and a constantly changing product offering, regular master data checks are essential. There, too, companies should think about how regular checks can be implemented with little effort and how adjustments can be processed quickly and efficiently. If you are a supplier yourself, it would also make sense to proactively communicate master data changes to your customers and send out regular master data overviews for review.