Ensuring high delivery capability in the product range with as little capital commitment as possible – a cost-effective AI-supported methodology opens up new possibilities
High delivery readiness usually costs a lot of money. Especially if there is no continuous readjustment. With a clever key figure and a modern optimization tool, a lot of money can be saved in technical retail despite high delivery readiness.
A high level of delivery readiness is crucial for a company’s competitive position in the technical trade. The best thing would be to always have sufficient stock of every item at all times. Unfortunately, delivery readiness also costs money in the form of high inventories; inventories that not only tie up liquidity but also cause running costs.
According to analyses by Abels & Kemmner, annual running costs of 19% to 30% of the portfolio value must be expected. Every €100,000 more stock then quickly costs €19,000 to €30,000 a year in running costs. This makes it clear that it is not only too little readiness to deliver that costs revenue, but also too much.
From a technical point of view, the high stock levels are due to the fact that the higher the supply availability of a product and the more fluctuating its demand, the higher the so-called safety stocks are required. If the item in question is also very expensive, the amount of safety stock quickly becomes a pain.
Sustainable strategies are a must
In our technical trade projects, we come across two prototypical strategies for ensuring delivery readiness. On the one hand, safety stocks are set very freely according to the gut feeling of the planning department or the sales department. The readiness for delivery of an article is therefore purely coincidental. If customers complain, the stock is simply increased a little. As customers never complain about excessive delivery capacity, stocks are rarely reduced. This strategy is dangerous. This can lead to a situation where, despite unnecessarily high stock levels, you do not achieve the delivery readiness required to keep up with the competition because the wrong items are in the wrong stocks.
The second prototypical strategy we observed makes much more sense. It is sometimes very difficult to calculate which delivery readiness leads to which stocks for which articles or article groups. Based on the consideration of what delivery readiness is required for certain article groups and what stocks are necessary for this, an attempt is made to find a compromise between delivery readiness and stock by distributing the delivery capability differently across the product portfolio. This is used to calculate the required safety and total stocks for the items. This procedure is complex and requires some theoretical knowledge. This is why the “operating point” once reached is often maintained.
The cost-benefit ratio must be right
Unfortunately, this approach still falls short. Once the desired delivery readiness across the offered product portfolio has been determined and the safety stocks have been set accordingly, not only must the safety stocks be readjusted monthly, but the delivery readiness levels should also be checked monthly. Changes in the demand behavior of the articles may require other safety stock levels in order to achieve the previously targeted delivery readiness. And due to changes in sales volumes and production costs of the items, it regularly happens that the delivery readiness previously targeted for one group of items becomes expensive, while it becomes cheaper for another group of items. For economic reasons, it therefore makes sense not only to readjust the safety stocks, but also to adjust the delivery readiness levels for such article groups.
As important as this approach is from an economic and competitive perspective, who goes to this effort in practice? Very few! It is therefore necessary to break new ground in order to find not only an effective, but also an efficient approach to optimizing the delivery readiness of a product portfolio. Ideally, it should be possible to readjust the readiness for delivery of a product portfolio within a few minutes so that a high level of readiness for delivery is achieved with the lowest possible stock levels. This is now possible thanks to the use of artificial intelligence (AI for short).
Artificial intelligence unties the Gordian knot
The starting point for the use of AI is the specification of a value-weighted overall delivery readiness of the product portfolio. This value indicates the percentage of the total value of customer orders for items in stock that you want to be able to ship on time, i.e. on the customer’s requested date or alternatively on the agreed delivery date.
The entire product portfolio in stock is then sensibly divided into ABC/XYZ classes; however, any other groupings are also possible. If specific AI-supported optimization algorithms are now used, such as those provided by the DISKOVER Service Level Booster developed by SCT GmbH, the delivery readiness levels for the defined portfolio fields are determined in such a way that the specified value-weighted overall delivery readiness can be achieved with the lowest inventory.
An optimization range can be assigned to the optimization algorithm for each group of the product portfolio to be considered, so that strategic considerations regarding the minimum and or maximum readiness for delivery of certain goods or groups of goods can be taken into account.
The delivery readiness levels determined can then be transferred to the ERP system. If this is not able to calculate reliable safety stocks required for a specified target readiness for delivery, the safety stocks can also be transferred directly to the ERP system.
It would not be possible to achieve the same degree of fine-tuning using classical mathematical methods, as the calculations required would be so extensive that it would take many billions of years (not a misprint) to calculate with today’s computing power.
Effective and efficient at the same time
The AI-supported optimization run of the Service Level Booster, on the other hand, takes a few minutes at most; 90 seconds is usually enough. Thanks to the simple procedure and the short calculation time, it is now also easy to readjust the target readiness for delivery in the portfolio fields on a monthly basis so that the target overall readiness for delivery can be achieved sustainably with low inventories. The achievable optimization potential naturally depends on a company’s initial situation. In applications where the distribution of delivery readiness in the product portfolio had not already been extensively optimized, safety stocks have already been reduced by up to 13% on average and overall delivery readiness increased by up to 16% at the same time (see Fig. 1). For article portfolios whose readiness for delivery has already been optimized with the help of APS (Advance Planning and Scheduling) systems, a further average reduction in safety stock of 3-5% was achieved.
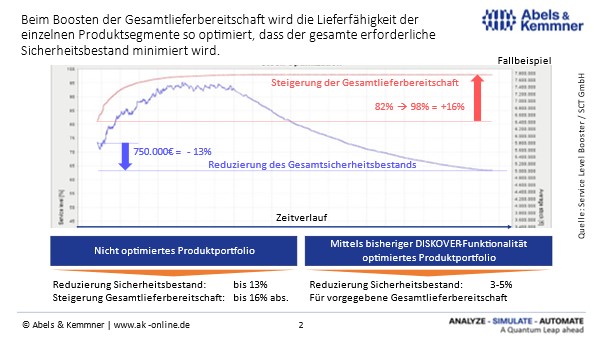
The quintessence
Adjusting the delivery readiness of your own product portfolio is only the spearhead of a comprehensive optimization of the entire planning process. Without a properly sharpened readiness to deliver, however, the market can hardly be hunted.