If I don't know what to do, do I make an AI right away? Expectations of AI in sales forecasting are often exaggerated
Many managers and administrators naively believe that artificial intelligence (AI) can finally provide accurate forecasts.
Instead of looking at historical data, it is better to rely on AI.
However, the reality is very different.
In reality, an AI system also learns from the past.
The AI may be able to recognize regularities beyond trends and seasonalities in the existing historical time series that traditional statistical forecasting methods do not recognize.
However, the ARIMA method is already very good in this respect.
Like any statistical approach, the ARIMA method does not reveal which specific factors influence the forecast.
However, an AI cannot do this either if it is only provided with historical time series from customer orders or outgoing stock data and no separate information on influencing factors that have had an impact on historical demand behavior.
If you have(prerequisite 1) assumptions about which external factors could influence market demand, you can go one step further and take these into account very efficiently and easily in AI-supported forecasts; especially if there are several factors that interact.
However, AI cannot find possible influencing factors on demand behavior independently.
At best, you can use large language models such as GPT (“ChatGPT”) to make assumptions about which influencing factors could have an effect if you have no assumptions yourself.
In order to quantitatively assess the effects of the assumed influencing factors on the market demand for a product or product group and to be able to make statements about future demand behavior, the AI also requires corresponding historical series of figures(prerequisite 2) on these factors with which the AI can be trained.
However, the fact that such historical data is available is not enough.
A sufficient amount of data must be available(requirement 3) in order to be able to train the AI.
How much data is required depends, as is often the case, on the individual case.
To put it pragmatically and unscientifically: 100 data sets are not enough, 1000 would be better, a few tens of thousands would be best.
In many cases, however, it is not possible to find that much data.
Furthermore, data on the influencing factors must also be available for the future(requirement 4a).
Only if you know how the influencing factors will change in the future can you determine the effects of this change on market demand.
Where this is not the case, there is still a chance(prerequisite 4b) that the current and historical data of the influencing factors already contain a statement about the future development of demand.
This sounds complicated, but it is quite simple: if, for example, the AI were to recognize from the training data that an increase in an economic factor, e.g. the IFO index or the purchasing index, leads to an increase in demand with a six-month delay, knowledge of the current value of these indices is sufficient to derive a statement about future demand in six months’ time.
In fact, some of our analyses from customer projects show that certain constellations of several economic factors together for certain product groups of certain customers make it possible to predict the development of demand for these product groups over the next three to six months.
As an example to illustrate this, let’s take the influence of competitor sales promotions on demand for a company’s own products.
In the case of standard products that are offered by different companies and that can replace each other, this is often a significant factor influencing the market demand for your own product.
Gathering information about competitors’ sales promotions can be very difficult, if not impossible.
At the very least, it would be necessary to know the dates of promotions and sales prices, and ideally also the sales volumes.
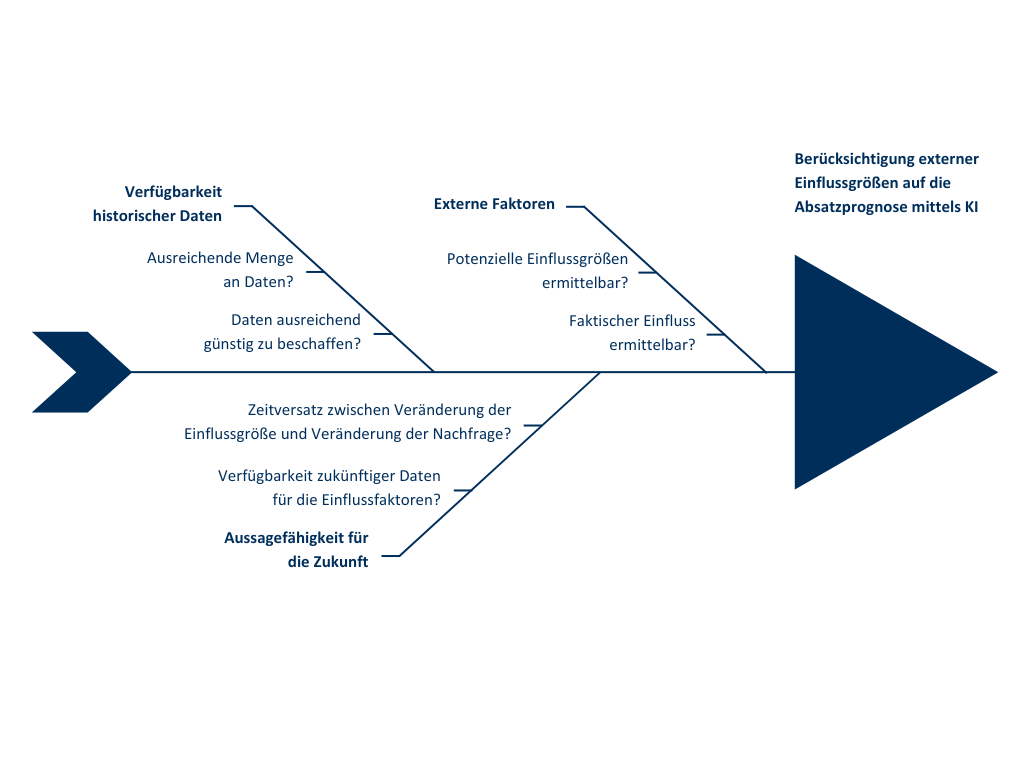
Five events (actions) can hardly be used to determine anything reliable.
You need to have knowledge of a sufficient number of events.
The weaker the impact of competitors’ actions on the demand for your own products, the more events are required to make statements with a reasonable degree of certainty.
If sufficient data is available and the AI can recognize an influence on the market demand for your own products with the existing data, this is already a big step, but is of no use if you do not know the future actions of the competition in advance.
Once the event has occurred, it is usually too late and the forecast is no longer of any use.
If competitor actions influence the future demand for your own products, a current action by a competitor can possibly lead to a temporary slump in demand for your own products.
However, this realization is unlikely to have an impact too far into the future.
Overall, it can be said that expectations of AI in terms of improving forecasts are still exaggerated, at least at present.
Although AI is able to learn from historical data and possibly recognize regularities that traditional statistical forecasting methods do not, there are still many challenges and limitations that need to be overcome.
Today, these no longer lie fundamentally in the possibilities of AI, but very often in the lack of data.
Missing data, which is worth a lot of money to those who have it and which is therefore increasingly rarely made available generously and free of charge for training AI systems…