Forget gut feeling – how to make better decisions with AI
Why your Forecasts should be smarter than your gut feeling
When intuition leads you astray
Jürgen was an experienced demand planner in a medium-sized company. With almost 20 years of experience, he had developed a good instinct for market developments. His colleagues relied on his judgment because his assessments were usually correct.
In the fall of 2021, however, Jürgen made a momentous mistake. In an important situation meeting, he argued in favor of significantly increasing production of a certain household appliance. “The market is picking up, I can feel it,” he explained with conviction.
What happened next fundamentally contradicted his Demand Planning: due to the global shortage of semiconductors, manufacturers of high-quality smart household appliances were unable to increase their production as planned. Ironically, this bottleneck led to consumers postponing their purchases instead of switching to simpler models – they preferred to wait for the availability of the scarce premium devices. However, Jürgen had predicted exactly the opposite and massively ramped up production of simpler devices. Instead of the expected sales boom, the company was left with an oversized stock. The financial losses amounted to several hundred thousand euros.
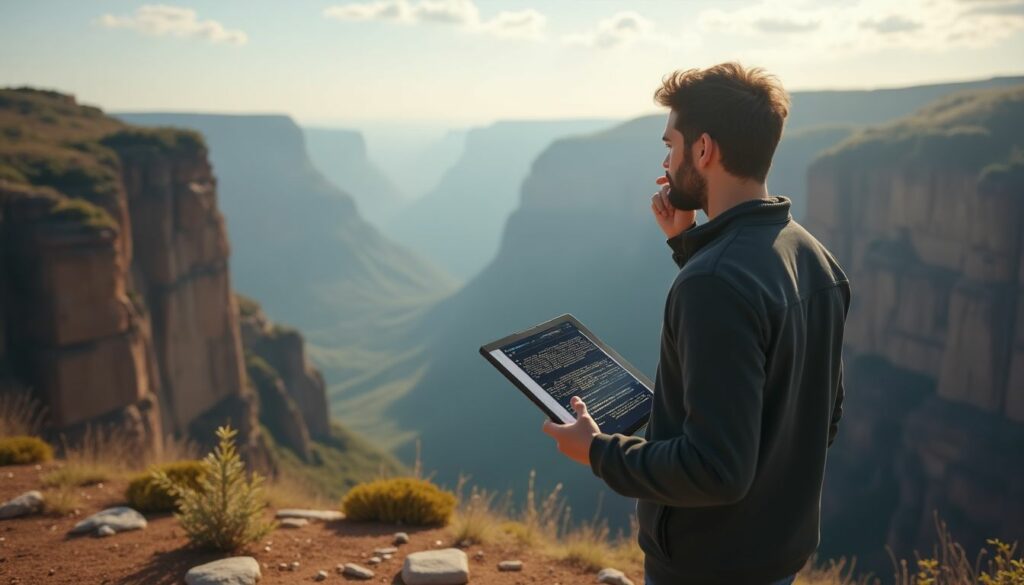
1. the limits of intuition in complex decisions
“My gut was actually always right” – this statement is often heard from experienced decision-makers. However, cognitive psychology shows that our brains are subject to systematic biases when making complex decisions.
Nobel Prize winner Daniel Kahnemann has identified two systems of thought in his research:
System 1: Intuitive thinking
This system works quickly, automatically and largely unconsciously. It is our constant companion in everyday life and processes information almost effortlessly. System 1 reacts immediately to stimuli, recognizes patterns based on previous experiences and generates emotional reactions. This is where our first impressions, spontaneous judgments and the much-cited “gut feeling” arise.
In practice, experienced Demand Planners use their System 1 when they immediately “feel” whether a forecast is plausible based on many years of experience. This intuition can be valuable – it is based on implicit knowledge that has been built up over the years. For example, an experienced Purchasing Manager often recognizes seasonal anomalies in sales data at first glance or senses when certain trends do not match the usual market behavior.
The strength of System 1 lies in its speed and efficiency. It enables us to react quickly in complex situations without having to consciously analyze every variable. This is particularly helpful in situations that are familiar to the expert and where patterns can be recognized.
System 2: Analytical thinking
In contrast, System 2 works slowly, methodically and requires conscious effort. It is our analytical thinking that proceeds logically, systematically evaluates data and weighs up probabilities. This system is active when we perform complex calculations, run through different scenarios or systematically weigh up the pros and cons.
System 2 is evident in planning and Demand Planning when teams carry out data analyses, evaluate time series, interpret market research results or create simulations of various scenarios. This thought process is more strenuous and time-consuming, but leads to more informed decisions, especially in the case of complex or novel problems.
The special quality of System 2 lies in its ability to deal with uncertainty, to understand statistical correlations and to proceed methodically. It can consciously question assumptions, weigh up different factors and systematically integrate new information. While System 1 reacts to known patterns, System 2 can develop new solutions.
The interaction of both systems
In practical application, both systems work together, with System 1 being constantly active, while System 2 is only switched on when required. Kahnemann describes this relationship as the “effortless generation of thoughts and impressions” (system 1) versus the “monitoring and control of thoughts and actions” (system 2).
For decision-makers in supply chain management, the balance is crucial: the intuition of an experienced Planner can provide valuable clues, but should be supplemented by systematic data analysis and modeling. The trick is to use both ways of thinking as appropriate to the situation – and to recognize when the gut feeling should be checked by analytical thinking.
When making forecasting decisions, these three psychological effects in particular influence our judgment:
• Overconfidence bias: The study by Dunning and Kruger (1999) showed that people often overestimate their own abilities. Participants in the lowest performance quartile assessed themselves particularly unrealistically – they saw themselves in the 62nd percentile, although their performance only placed them in the 12th percentile.
• Loss aversion: People perceive losses more intensely than equivalent gains. This asymmetry means that many companies prefer to hold too much Safety stock out of fear of bottlenecks and underestimate capital costs and depreciation risks.
• Confirmation bias: We tend to favor information that confirms our existing beliefs. Studies have shown that people are about twice as likely to look for information that confirms their existing views instead of looking for contradictory evidence, which has a significant impact on the quality of decision-making in a business context.
These cognitive biases not only influence individual decisions, but also shape organizational culture and thus the way Forecasts are made. While experienced Planners bring valuable tacit knowledge to the table, research shows that systematic data analysis can compensate for these human weaknesses.
This is where modern forecasting methods come into play – but even the classic approaches are reaching their limits in today’s dynamic economic world, as we will see below.
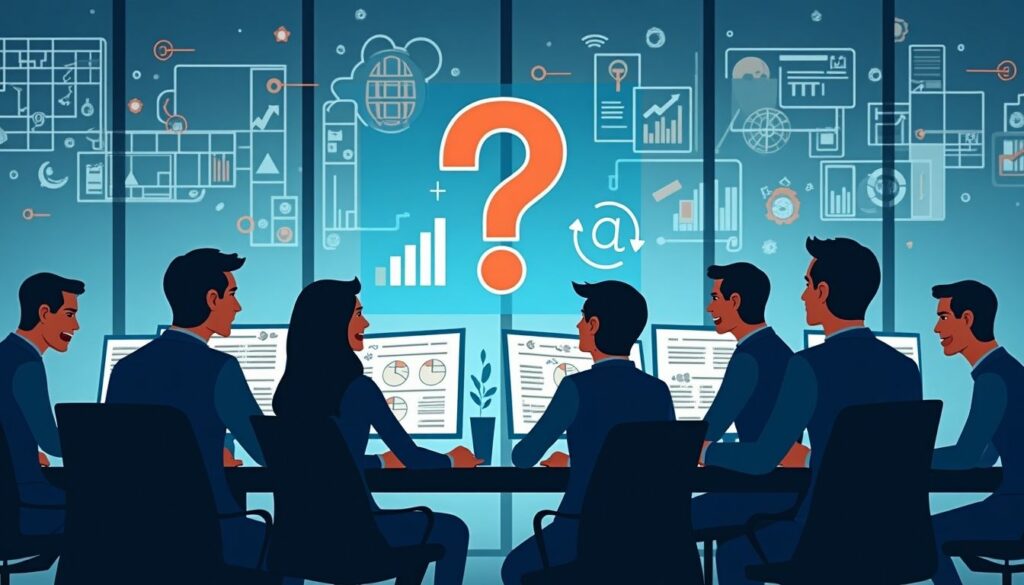
2. why classic forecasting methods are reaching their limits
Classic Forecast procedures such as moving averages, exponential smoothing and seasonal decomposition methods have been the standard tool for Demand planning for decades. They provided companies with reliable services in times when markets were more predictable and changes were slower. However, in an increasingly dynamic and networked business world, their limitations are becoming ever more apparent and painful:
Past orientation – driving with a view only in the rear-view mirror
Traditional forecasting models are based on a fundamental principle: they analyze historical data and project identified patterns into the future. This approach is like trying to steer a car while looking only in the rear-view mirror – it works reasonably well as long as the road is straight and there are no unexpected obstacles.
However, in a world full of “curves” and “obstacles”, this method is becoming dangerous. Global supply chain disruptions, disruptive technologies, changing consumer habits and unpredictable events such as pandemics or geopolitical conflicts mean that historical data is less and less predictive of future developments.
A drastic example from the real world: consumer behavior changed fundamentally during the COVID-19 pandemic. According to GfK, sales of webcams and monitors in Germany rose by up to 81% in just a few weeks – a leap that conventional forecasting methods could not have predicted. At the same time, demand for business clothing plummeted by more than 45%. Companies that relied solely on historical data were either sitting on empty warehouses or unsaleable stock levels.
Classical methods could never have predicted such a slump or rise, as such patterns simply did not exist in the historical data. The pure focus on the past was transformed here from a methodological strength into a dangerous weakness that cannot be compensated for without a good dose of common sense.
Other significant disadvantages of classic approaches:
Limited ability to recognize complex relationships: Traditional forecasting methods can generally only map linear or simple non-linear relationships. They work according to the principle of isolating individual variables and their direct influences. However, the reality of modern economic activity has a much more complex structure, with nested interactions and multi-level dependencies between numerous factors.
These models reach their limits when it comes to capturing synergy and cannibalization effects or identifying thresholds at which certain factors suddenly become relevant. A typical example: a classic forecasting model is relatively good at predicting how a price reduction will affect demand – but it fails to understand how this price reduction in combination with a competitor promotion, unfavorable weather and a viral social media trend will affect it simultaneously. It lacks the ability to model the complex orchestration of different influencing factors and take their non-linear interactions into account. Especially in food retail, understanding such dynamics can be very helpful for short-term Requirements planning.
Difficulties in integrating external data sources: Traditional models typically work with a limited number of carefully selected internal variables – sales history, prices and perhaps promotion calendars. But in a networked world, the decisive influencing factors often lie outside the boundaries of the company. The integration of large volumes of heterogeneous external data overwhelms these systems in several ways.
On the one hand, they often lack the methodological flexibility to process unstructured data such as customer ratings, competitive activities or social media sentiment. Secondly, they fail due to the sheer volume of data: while an AI system can easily analyze hundreds of potential influencing factors, traditional models require the relevant variables to be pre-selected manually – often overlooking crucial factors. And last but not least, the computing power of traditional procedures also reaches its limits when real-time data from a wide variety of sources – from weather sensors to online clickstreams and macro indicators – is to be continuously incorporated into Demand Planning. Yet it is precisely this broad database that is often the key to understanding complex market dynamics.
Limited adaptability to change: Conventional forecasting methods show a structural inertia that is hardly noticeable in stable markets, but has serious consequences when disruptive changes occur. This inertia arises from the focus on long-term data patterns and the inherent assumption of a certain stability. In the event of sudden market changes – whether due to new competitors, changing consumer preferences or external shocks – this leads to a problematic delay in reaction.
An example illustrates the problem: after an abrupt change in customer behavior, conventional models will initially interpret the new data as “outliers” and largely ignore it. Only when the new pattern stabilizes over several periods do these systems slowly begin to adjust their parameters. Depending on the model, this adjustment period can take weeks or months – a period in which the Forecasts deviate systematically and significantly from reality. By the time the system has fully integrated the “new normal” into its forecasts, costly mistakes have often already been made – whether due to excessive stock levels or inability to deliver.
The answer to these challenges: AI-based Forecasts
In view of these fundamental limitations of traditional forecasting methods, more and more forward-looking companies are turning to more modern approaches. Artificial intelligence and machine learning offer ground-breaking opportunities that overcome the limitations described above. The following section shows exactly how AI-based systems can revolutionize Forecast accuracy by recognizing complex patterns, integrating external data and adapting quickly to changes.
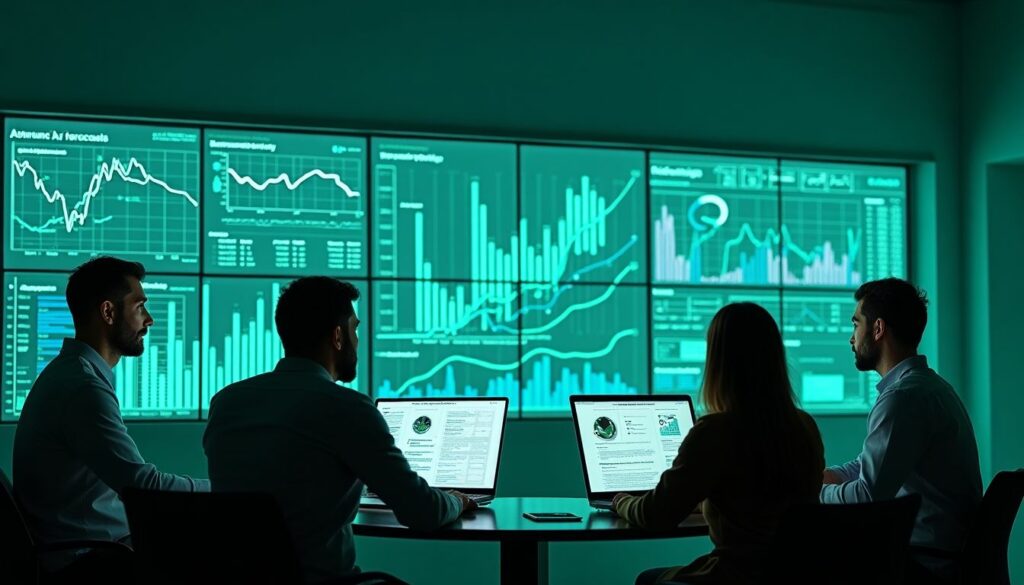
3. how AI-based Forecasts can improve accuracy
Artificial intelligence and machine learning are revolutionizing Demand plan with capabilities that go far beyond traditional statistical procedures. The fundamental difference lies in the way these systems handle data and correlations:
Recognition of complex, non-linear relationships: While traditional models are usually based on linear relationships or predefined patterns, AI systems can identify and map highly complex, non-linear dependencies. This is comparable to the difference between a simple city map and an intelligent navigation system that takes traffic flows into account in real time.
Deep learning architectures such as multi-layer neuronal networks are able to recognize hidden patterns that would not be visible to the naked eye or traditional algorithms. For example, an AI system can recognize that the demand for a product does not simply rise or fall in proportion to the price, but that this relationship depends on factors such as the day of the week, weather, season and even the placement of competing products. This ability is particularly valuable for products with complex demand patterns such as seasonal goods, fashion products or items that are highly dependent on the weather.
Processing large, heterogeneous amounts of data: The strength of modern AI systems also lies in their ability to integrate and analyze enormous amounts of data from a wide variety of sources – something that would be virtually impossible to do manually or using traditional methods. An advanced forecasting system can simultaneously process internal company data, competitive information, social media trends, weather data, macroeconomic indicators and industry-specific key figures.
The ability to use unstructured data is particularly impressive: While classic procedures rely on neatly formatted series of numbers, modern AI systems can extract valuable information from customer reviews, forum posts, news articles or even images. For example, a fashion company can use AI to analyze social media sentiment on certain trends and incorporate this information into the sales forecast at long before these trends are reflected in the sales figures.
Continuous adaptation to new market conditions: One of the most revolutionary features of AI systems is their ability to learn. Unlike static models, which have to be recalibrated manually, AI-based forecasting systems can learn continuously and adapt autonomously to changing market conditions.
This adaptive capability is based on advanced techniques such as reinforcement learning and online learning, in which the system constantly checks the quality of its own Forecasts and adjusts its parameters accordingly. In the event of sudden market changes – whether due to seasonal effects, competitor actions or external events – a well-trained AI system can react much faster than conventional methods. It often recognizes new patterns after just a few data points and adjusts its predictions accordingly.
This adaptability is further enhanced by techniques such as anomaly detection (detection of unusual patterns) and change point detection (identification of trend changes). This allows AI systems to recognize changes in the market at an early stage and adjust Demand Planning accordingly, even before human analysts would notice these trends.
A practical example: a large German retail company implemented an AI-supported forecasting system that combined sales data with additional factors such as weather conditions, promotional activities and seasonal trends. The result was a 22% improvement in Forecast accuracy and a 15% reduction in stock levels while maintaining product availability (Handelsblatt, 2022).
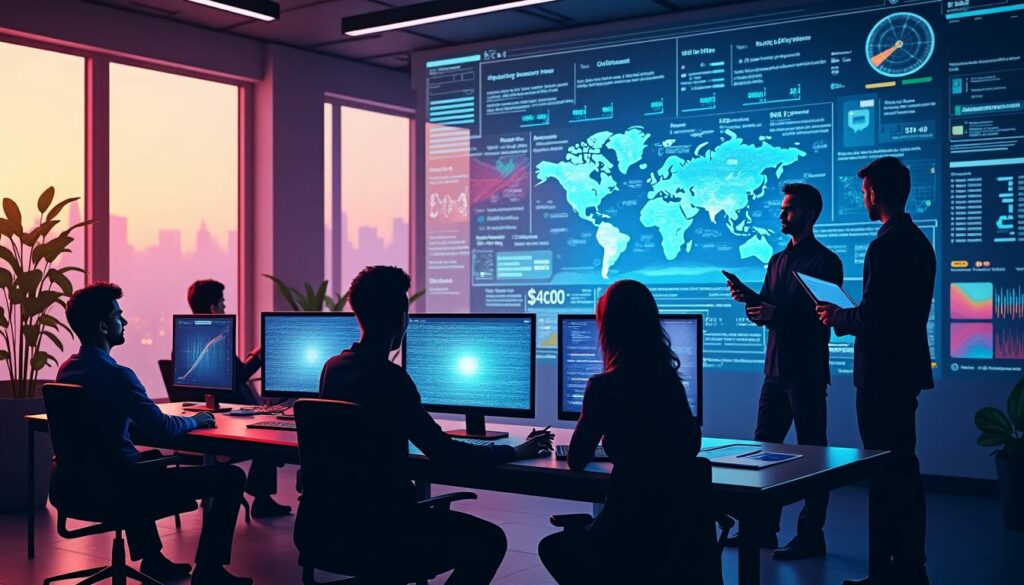
4. The power of external data in AI forecasting models
A key advantage of modern AI systems lies in their ability to integrate external data sources and analyze their influence on demand:
Social media trends: A recent survey by GetApp (2023) shows that 80% of consumers are influenced in their purchasing decisions when influencers collaborate with a particular brand. This effect is particularly strong in certain product categories: 40% of respondents who follow influencers on social media stated that they had bought fashion items after seeing them on influencers. The same applies to food (36%) and beauty products (32%). AI systems can capture these specific trends differentiated by product category and incorporate them into the forecasting models in real time.
Economic indicators and market data: The integration of economic data into sales forecasts can significantly improve the accuracy and reliability of these forecasts. By taking into account macroeconomic indicators such as gross domestic product (GDP), unemployment rate, consumer price index (CPI) or specific industry figures, companies can gain a more comprehensive picture of market dynamics. This data reflects the general economic situation and directly influences consumer behavior. For example, a rising unemployment rate can lead to a decline in consumer spending, while positive developments in industry-specific indicators such as production figures or incoming orders can indicate rising demand. AI systems are able to recognize these complex correlations between economic indicators and sales trends and use them for more precise long-term forecasts
Weather data: According to a study by UBIMET (2022), weather-based forecasting models can improve accuracy for certain product categories by 15-25%. The weather plays a major role in demand, especially in food retail with sufficiently short replenishment times. However, as soon as replenishment times become longer and the inaccuracy of the weather forecast is greater than that of the item itself, weather data can no longer improve the relevant Forecast accuracy.
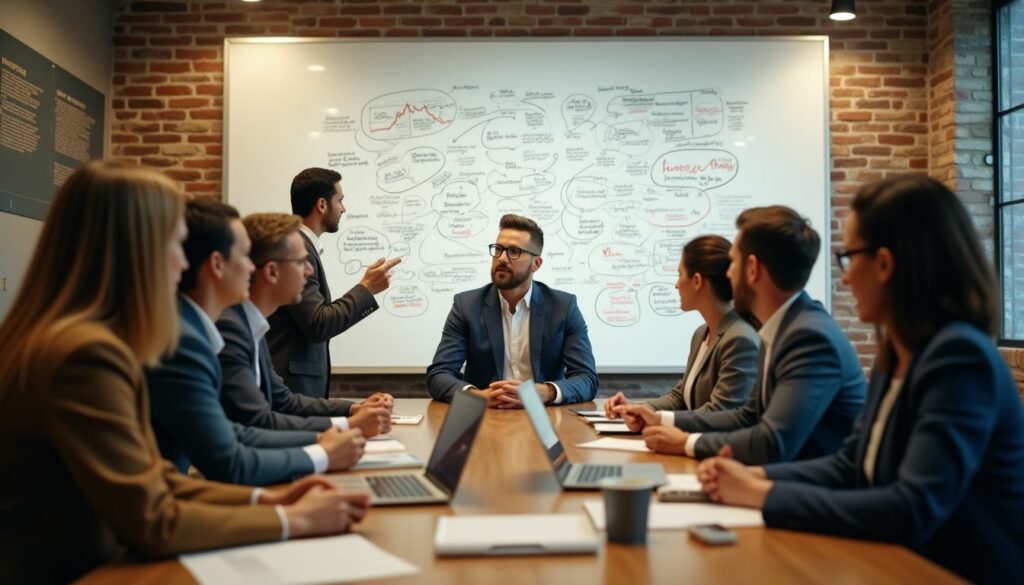
5. The added value of human expertise
Despite all the technological advances, there are situations in which human judgment remains indispensable:
For product launches and innovations: For new products without a sales history, even advanced AI systems come up against natural limits. The forecasting models simply have no database on which to learn. In such situations, the implicit knowledge of experienced product managers and market experts is irreplaceable. They can draw on experience with similar product launches, interpret market research data and incorporate qualitative factors such as customer feedback from test markets or early reactions from retail partners. A practical approach is the combination of AI-supported analysis of comparable products (known as product mapping) and structured collection of expert assessments. It has been shown that hybrid forecasts, which take into account both algorithmic components and expert judgment, are on average 30% more accurate for new product launches than purely data-driven or purely intuitive estimates.
In the event of unforeseen events and disruptive changes: Sudden, unprecedented events overwhelm any AI system that has been trained solely on historical data. The COVID-19 pandemic provided numerous examples of this: While algorithms continued to produce stable Forecasts due to a lack of comparative patterns in the past, human decision-makers quickly recognized the extraordinary situation and its potential impact. As a result, many companies reacted by manually adjusting their Order quantities – for hygiene products, home electronics or leisure items, for example. This ability to react quickly required human contextual knowledge, improvisation and the ability to link different sources of information. Experts who had experienced similar crisis situations in the past and their empirical knowledge proved to be particularly valuable. A leading food manufacturer reported that the information relevant to decision-making in the initial phase of the pandemic did not come from the forecasting systems, but from direct conversations with trading partners, suppliers and international colleagues who had previously been affected by the crisis.
Qualitative and structural market changes: Certain types of changes elude algorithmic recording because they are more qualitative than quantitative in nature. The impact of regulatory changes such as new environmental regulations, customs regulations or product standards can often hardly be derived from historical data. The same applies to the emergence of new competitors with disruptive business models or the entry of established providers into new market segments. In such situations, experienced experts with their industry knowledge, personal networks and ability to think laterally have an advantage. They are better able to assess potential effects because they can look back on comparable situations, anticipate stakeholder reactions and include non-linear effects in their considerations. A pragmatic approach here is to integrate structured expert assessments into the forecasting system, for example through regular “market radars” in which qualitative information on competitors, regulatory trends and technological developments is systematically recorded and evaluated.
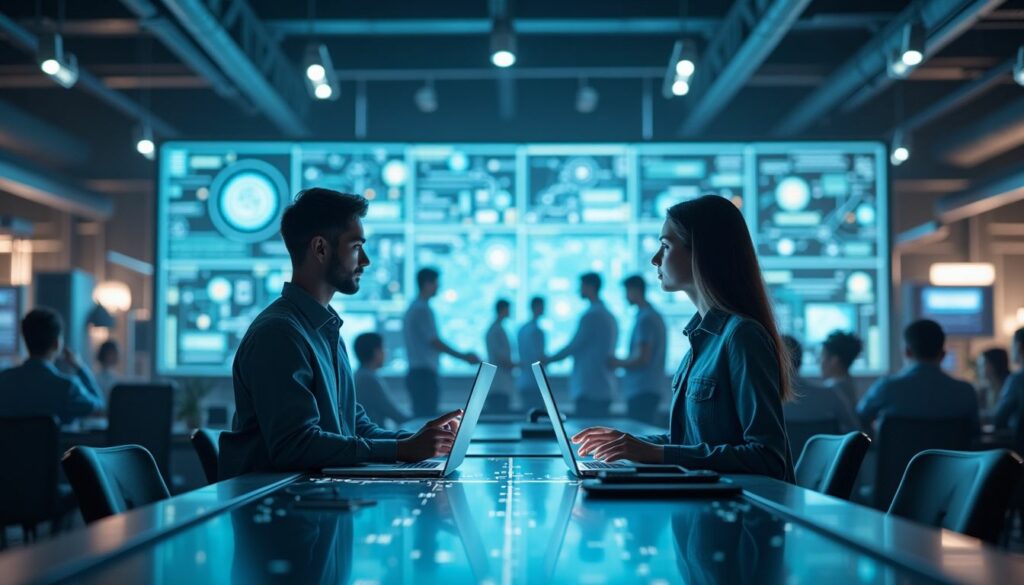
6. The optimal synergy between man and machine
Neither AI systems alone nor purely human decisions can achieve optimal results in today’s complex business world. The true added value only unfolds when companies specifically combine the complementary strengths of both worlds – the analytical power and data precision of artificial intelligence with the intuition, creativity and contextual judgment of experienced experts. This symbiosis enables Forecasts that are both data-driven and practical and realistic.
How can companies put this synergy into practice?
- Setting up a Demand Planning Board: Implementing a cross-functional team that meets regularly to evaluate and refine AI-generated Forecasts is a critical success factor. This board should include representatives from different departments – from sales and marketing to supply chain, production and finance. The different perspectives enable a holistic view of AI Forecasts. The integration of employees with direct customer contact is particularly valuable, as they are often aware of market changes at an early stage. A two-stage process has proven itself in practice: First, an automated plausibility check of the AI forecasts based on defined rules, followed by a targeted human review of conspicuous deviations. Companies such as Procter & Gamble have improved their Forecast accuracy by up to 20% using this approach.
- Continuous feedback and learning: AI models differ fundamentally from static algorithms in their ability to continuously learn and adapt. A structured feedback process is essential if this potential is to be fully exploited. This should not only record quantitative deviations between Demand Planning and actual requirements, but also qualitative information on the causes of these deviations. Timely feedback is particularly important in the event of unexpected market developments or special events such as product launches or advertising campaigns. An effective feedback system systematically documents which factors were responsible for forecast deviations – whether internal factors such as delivery problems or external influences such as competitor campaigns or unforeseen events. This information then flows into the calibration and further development of the AI model, making the system increasingly precise over time.
- Transparent decision-making processes and explainable AI: One of the biggest challenges in the acceptance of AI systems is the phenomenon of the “black box” – decision-makers are reluctant to follow recommendations whose origins they cannot understand . It is therefore crucial to rely on “explainable AI” approaches that make the decision-making paths of AI transparent. Visualized representations of the most important influencing factors for a particular Demand Planning, comprehensible weightings of different variables and the ability to delve into different levels of detail in the analysis have proven themselves in practice. Leading companies also offer their Planners a scenario function with which they can simulate the effects of various assumptions on the Demand Planning – for example: “How would the forecast change if competitor prices were to fall by 10%?” This transparency not only promotes acceptance, but also enables a more qualified evaluation of the AI recommendations.
- Comprehensive training and change management: The technical implementation of an AI system is only the first step – lasting success depends largely on the people who work with this system. According to a comprehensive study by McKinsey (2023), up to 70% of AI initiatives fail not due to technical problems, but due to a lack of user acceptance and insufficient understanding of the possibilities and limitations of the technology. A well-thought-out training program should therefore cover various levels: from basic concepts of AI and machine learning to the specific functionality of the system used and practical workshops in which employees practice using the new tools. Training courses that work directly on real use cases from the respective company context are particularly effective. In addition, it is advisable to appoint “power users” or “AI champions” in the specialist departments who act as multipliers and initial contacts.
Conclusion: The path to more precise Forecasts
The combination of proven statistical methods, AI-supported Forecasts and human expertise offers companies the opportunity to significantly improve their planning accuracy. A study by Gartner entitled “Predicts 2022: Supply Chain Technology” shows that companies using this hybrid approach were able to increase their Forecast accuracy by an average of 25%. The analysts point out in particular that the greatest improvements were achieved where all three approaches were brought together in a structured process (Gartner, 2022).
Jürgen, the demand planner from our initial example, has learned from his experience. Today, he uses a data-supported system with statistical procedures and AI forecasts as the basis for his Forecasts, but still contributes his valuable market experience – no longer as the sole basis for decision-making, however, but as a supplementary perspective.
If you want to learn how AI forecasting can benefit your business, join our online live session on 14 May 2025. Click here for free registration: AI Forecasting.
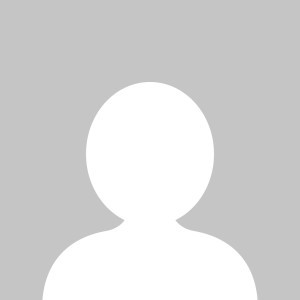