In the development of AI systems, there is a complex relationship between data quality and data quantity. For a long time, it was assumed that large amounts of data would automatically lead to better results. This misconception meant that the sheer volume of data was seen as a good indicator of the value of a data set.
In the meantime, awareness has changed significantly: The quality of the training data is crucial to the success of AI models. High-quality data enables the system to imitate human intelligence and decision-making more accurately. Several factors are decisive for data quality:
- Completeness of the data
Reliability and validity - Availability and timeliness
- Consistency and consistency
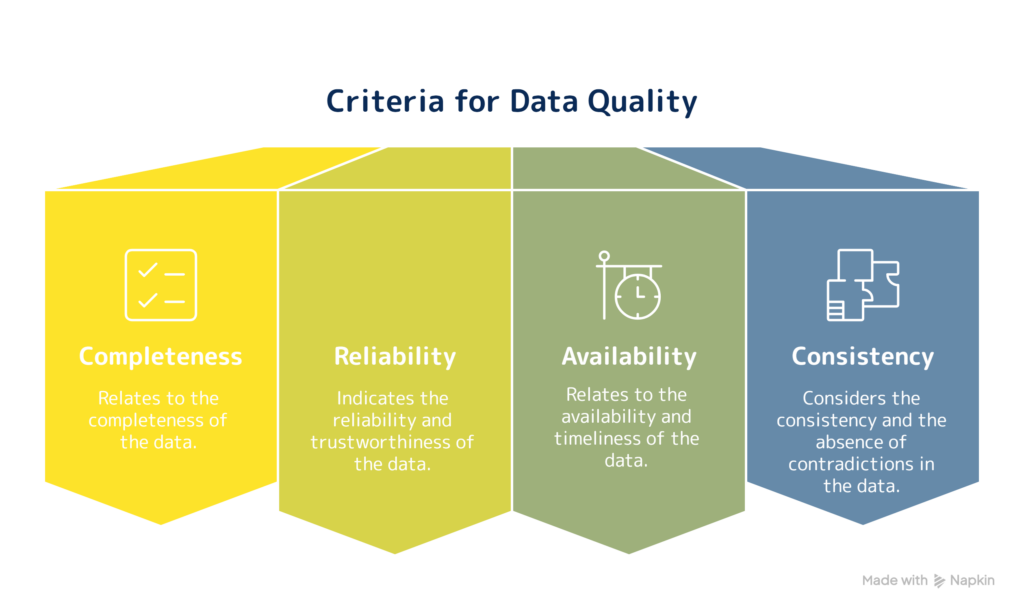
For large language models, the enormous amount of data was a decisive factor. These models were trained with huge amounts of data and corresponding computing power, which made it possible to compensate for certain quality deficiencies.
For small and medium-sized companies, however, data quality is the key to success. Good data quality means that less data is needed to achieve good results. This makes it possible to train smaller models with less effort, which also makes AI economically viable for these companies
The importance of data quality is demonstrated to us every day in our work. Whether we are training AI models for forecasting or filling our digital twin with ERP data for a supply chain management optimisation project, a considerable amount of time always goes into improving data quality and cleansing data.
Incorrect data cannot always be recognised at first glance! You have to have detailed knowledge of the field from which the data is obtained in order to be able to judge its quality. Errors can also occur because the data used does not completely cover the field of analysis. Data mass does not automatically mean data completeness!
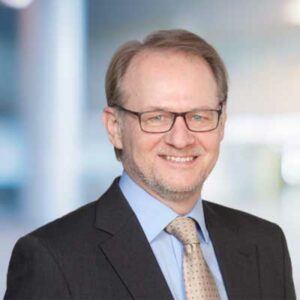