Artificial intelligence, real mistakes – why even AI-based forecasts can’t predict everything
Between wishful thinking and reality
Artificial intelligence is regarded as the key technology for demand forecasting of the future. In whitepapers, webinars and countless LinkedIn posts, it is being touted as the game changer in supply chain management par excellence: precise, adaptive, scalable. Who could resist?
Mr Schneider is Head of Demand Management in a medium-sized industrial company. For months, he has been working intensively on the possibilities of artificial intelligence in demand planning. On LinkedIn, in webinars and specialist articles, he reads and hears about impressive successes – we are talking about self-learning forecasting models that reliably recognise trends, compensate for fluctuations and deliver stable forecasts even for complex items.
And indeed, traditional statistical forecasting often reaches its limits in everyday life. Especially when it comes to the “problem children” in the product range – items with sporadic consumption, strongly fluctuating demand or abrupt structural breaks. For example, when a competitor product suddenly disappears from the market, or a customer changes their ordering behaviour from one day to the next.
The hope lies in AI forecasts: can they finally provide a remedy where statistical methods deliver unreliable forecasts?
But this is where the real story begins. Because even modern AI forecasts have limitations – not because they are “bad”, but because they are based on data, and data alone never tells the whole story. If you understand this, you can assess the potential of AI forecasts much better – and utilise them.
In this article, we show where AI forecasts reach their limits, why this is quite normal – and what you can do to get better forecasts anyway – with the right mix of classic demand planning, statistical methods and AI-based forecasting models:
- What are the typical stumbling blocks in AI forecasts?
- Which challenges are homemade – and which are systemic?
- And how can we compensate for weaknesses instead of suppressing them?
By the end, you will know why AI can’t do everything – but where it is used correctly, it can be a real asset.
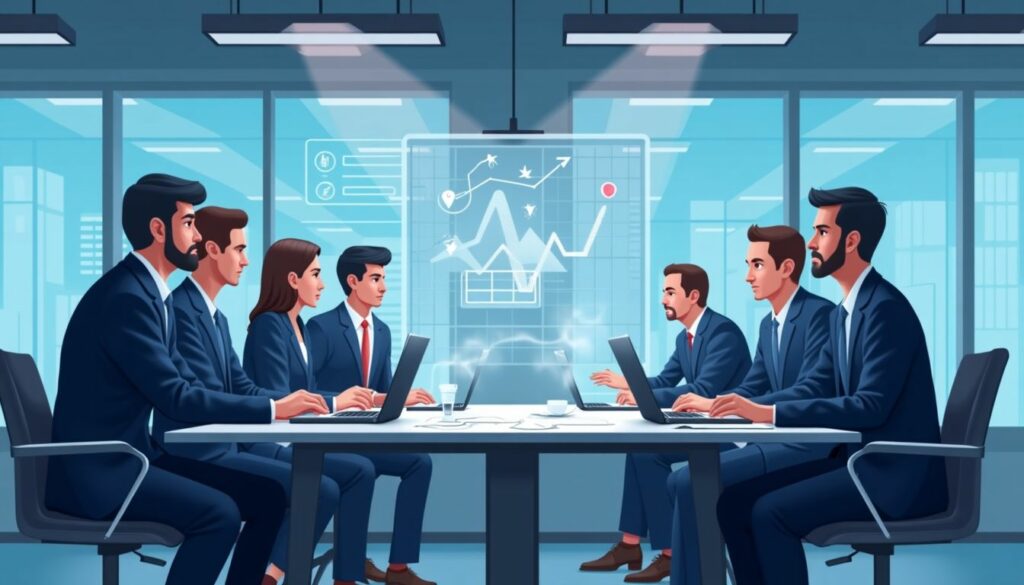
AI is not an oracle
Artificial intelligence promises a lot – and is often overloaded with even more expectations. In supply chain management we often hear: “With AI we have precise forecasts, automated forecasting, less planning effort – at last”. And it’s true: AI can do a lot. But what is often neglected is a sober look at the prerequisites – and the limitations.
Many articles, webinars and LinkedIn posts celebrate the success of AI-based forecasts, but little is said about the conditions under which these successes are even possible. The fact is that even AI cannot predict the future. It recognises patterns – no more and no less. In an international context, this is often referred to as demand forecasting, i.e. data-based demand planning with the help of intelligent processes. And only if these patterns exist somewhere in the historical data.
In contrast to traditional statistical methods, however, AI forecasts have a number of other advantages:
- It can include external data, e.. weather, public holidays, economic indicators.
- It continues to learn with each new data point and develops dynamically. It therefore continuously optimises itself – which can be a great advantage in modern forecast models, especially when using machine learning.
- It recognises more complex relationships, for example by classifying by product type, ABC/XYZ characteristics or other attributes.
But here, too, the following applies: only if the past provides a reliable indication of the future can AI derive meaningful forecasts from it. Without a high-quality, sufficiently large and relevant database, any AI – however modern it may be – is ultimately a paper tiger.
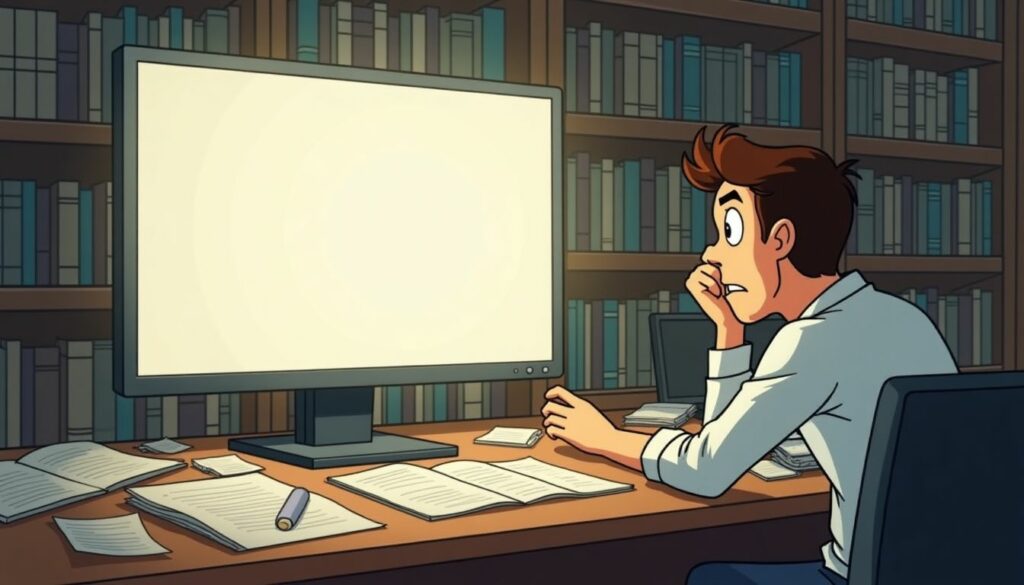
No forecasts without a solid foundation: Why sporadic articles remain a problem
Like any data-based process, an AI model depends on the quality and quantity of its input data. And this is precisely where one of the biggest problem areas becomes apparent in practice: many companies have a large number of items in their portfolio whose sales trends are simply too sporadic or too irregular to derive a reliable forecast.
A typical example: an item that has only been sold on two or three occasions in the last twelve months. Perhaps because it is only ordered when needed. Perhaps because it has a very specific application. For humans, this behaviour is somewhat plausible – for an AI model, however, it remains a mystery.
The challenge here is that a model can only learn if it recognises a certain regularity or at least statistically usable patterns and, above all, has enough data points. However, in cases like these, the data is simply too thin. And this doesn’t just apply to AI – traditional statistical forecasting methods such as exponential smoothing or moving averages also fail here. By using external factors such as economic indicators or adding features as additional data inputs, AI may be able to deliver reasonably useful forecasts earlier, perhaps as early as six months of consumption in the last 12 months instead of eight, but at some point, even AI will run out of fun.
What is often overlooked: AI is not a miracle machine that fills the gaps with magical intelligence. It is not a fairy by the wayside that can magically fill data gaps with its magic wand. If you feed in too little or too inconsistent data, you won’t get reliable results even with the best algorithm. The belief that “AI will sort it out” is a fallacy – at least if the necessary data feed is missing.
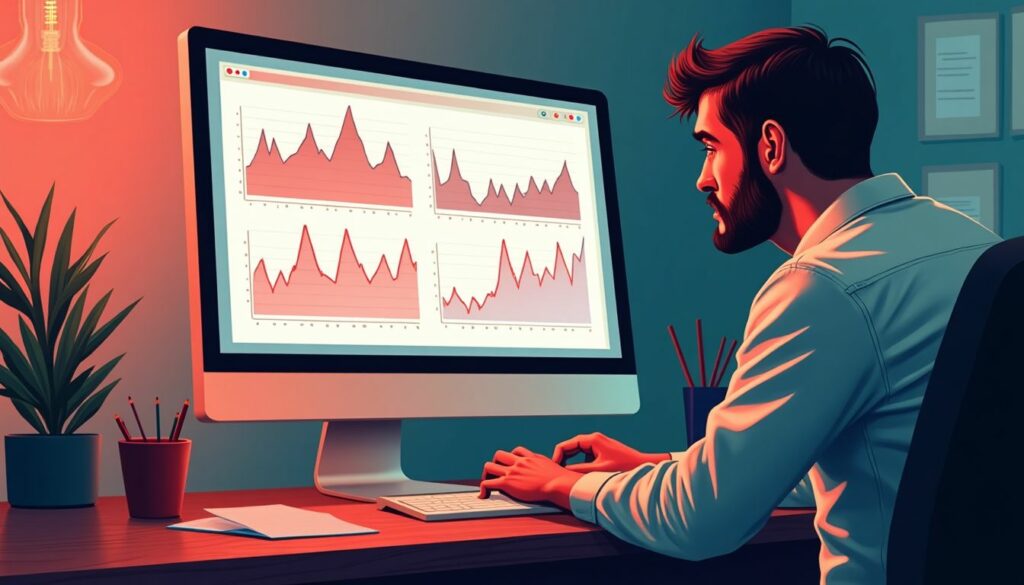
When consumption knows no pattern – and the algorithm has no chance
Some products behave like wild horses: they simply cannot be tamed – neither by traditional forecasting methods nor by modern AI. Their consumption fluctuates seemingly at random, the demand patterns are unclear and the influencing factors are diffuse.
A practical example: an item that is hardly in demand in the first quarter, then suddenly experiences a peak in June, drops off again in July – and unexpectedly takes off again in October. Without external clues, as a special promotion, a large order or a seasonal effect, this dynamic remains a mystery for the model.
The situation becomes even more critical in the event of structural changes: A competitor’s product suddenly drops out of the market, a new customer regularly orders larger quantities, or a change of supplier alters ordering behaviour. These are all changes that can neither be announced in the history nor “learnt” by the algorithm in advance.
This reveals a key weakness of data-driven systems: they are reliant on patterns that have manifested themselves in the past. However, if the system environment changes abruptly, the foundation is missing.
As a result, even the best AI model cannot reliably anticipate such disruptions. Without contextual information – i.e. without the human ability to categorise market developments – the forecast remains a shot in the dark.
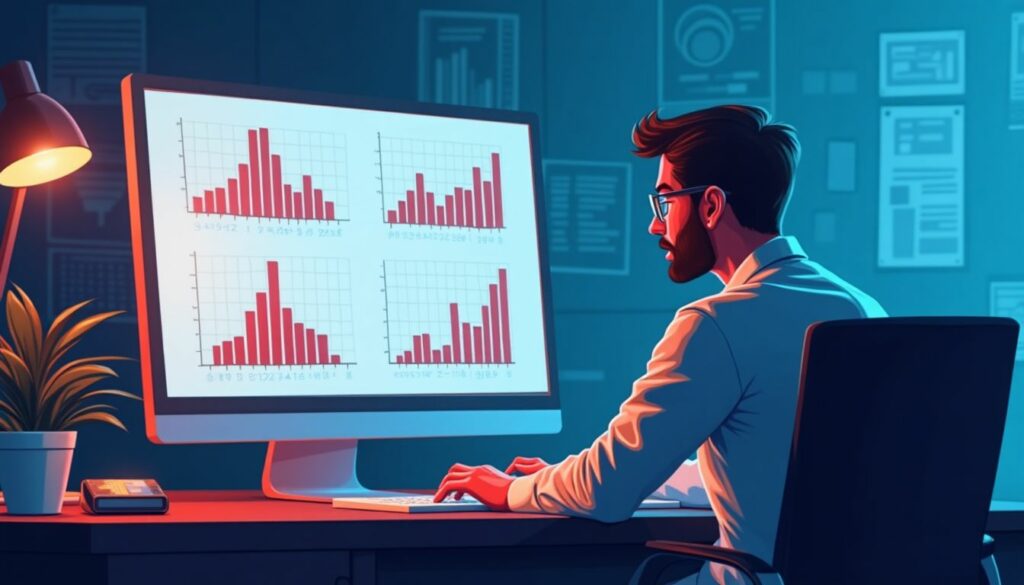
How incorrect parameters and poor data sources can distort forecasts
As powerful as AI models may be, they are not immune to poor foundations. An incorrect setup, faulty data or unsuitable parameterisation can quickly turn a promising forecasting model into a below-average provider of results.
In everyday life, we repeatedly encounter typical sources of error that occur much more frequently in practice than you might think:
- Distorted historical data: Special offers, promotions or one-off events are often not recorded or labelled correctly. The pandemic years or crisis phases in particular can massively distort the history without the model “knowing” this.
- Incomplete data sets: Missing time periods, sales channels or gaps due to system changes are poison for any forecasting model – whether traditional or AI-based.
- Overfitting or underfitting: If the model is trained on too many or too few, but very specific items, it can either react too sensitively (overfitting) or too superficially (underfitting) – neither of which is very helpful in practice.
- Incorrectly aggregated data: If forecasts are not made at individual item level (e.. SKU) but at higher aggregated levels, important differences are lost. A classic example: everything appears stable at product group level, but chaos reigns at SKU level. And this chaos flows directly into production via production planning, which inevitably takes place at SKU level.
- Unmaintained master data: Outdated article information, in particular incorrect categories, lead to errors – especially in feature engineering, i.e. working out relevant influencing variables.
What helps? Consistent, structured data quality management. And above all: close cooperation between demand planning and master data management. After all, quality assurance is not a purely technical discipline – it is an interdisciplinary responsibility.
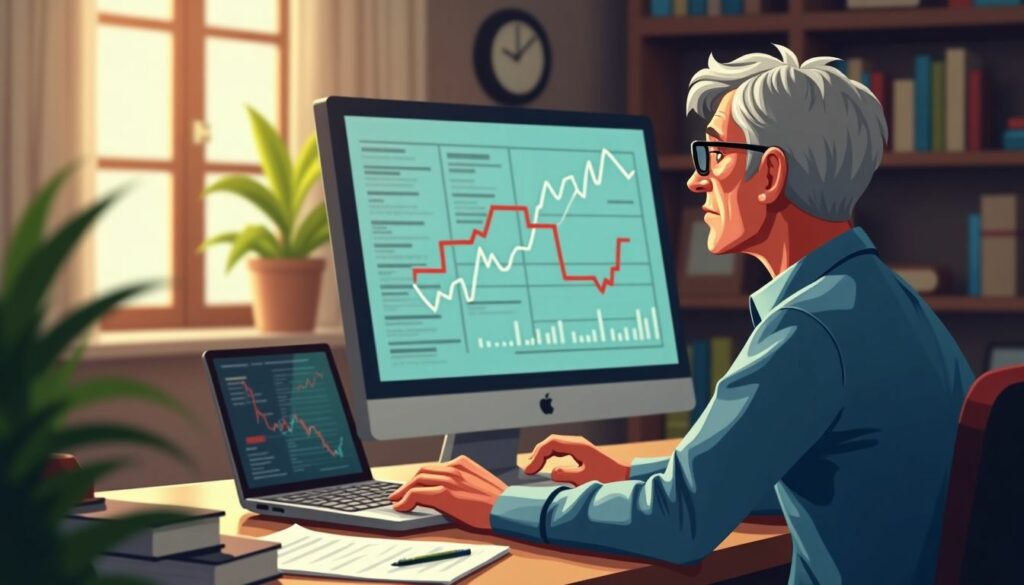
Why experience, intuition and market knowledge still count
As much as AI can do, it does not (yet) have any intuition. It doesn’t understand irony, doesn’t know market rumours and doesn’t read customer emails between the lines. But that is precisely what makes experienced planners so valuable.
A practical example: An AI model forecasts a significant decline for an item because there has been little movement in recent months. However, the planner knows from discussions with the sales department that a new framework agreement is about to be signed. The supposedly poor sales forecast will soon be a waste of time – if nobody intervenes in time.
AI can do a lot, but it needs control. It is not an autopilot, but a co-pilot: powerful, fast, analytical – but not infallible. Especially in the case of outliers, one-off market events or products of strategic importance, the interaction between man and machine is crucial.
The best forecasts are created where those responsible for planning understand the AI, critically reflect on its results and override them if necessary. Because only those who know the system can use it sensibly.
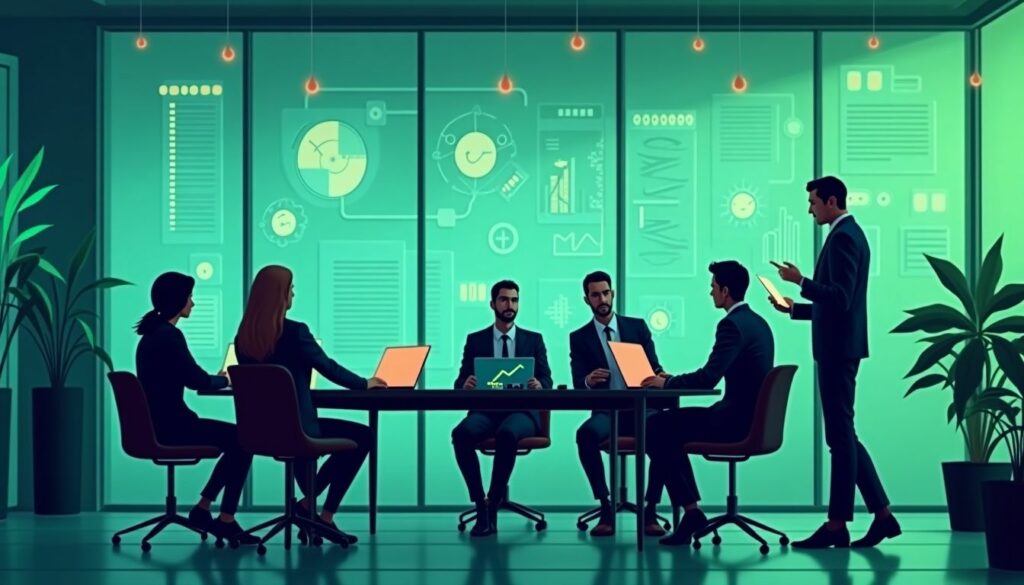
How to get the best out of your AI forecasts
The first step towards more stable forecasts is to recognise sources of error at an early stage. Many problems can be avoided if you regularly take the time to check the models, critically scrutinise their results and deliberately avoid falling into an automated mode. After all, if you simply run forecasts without reflecting on them, you risk perpetuating incorrect assumptions – be it through outdated data, unconsidered outliers or external effects that are simply not reflected in the model.
Monitoring AI models is not an end in itself either. It’s not about monitoring the machine – it’s about understanding what makes it tick. Interpretation becomes a duty, not an optional extra.
And finally: the best results are always achieved through teamwork. Man and machine complement each other – but only if both are allowed to contribute their strengths.
📌 Mini checklist – 3 levers for better AI forecasts:
- Actively ensure data quality:
Regularly check whether your input data is complete, correct and consistent. This applies not only to sales data, but also to master data such as product types, customer allocations or product-specific characteristics such as life cycle phases or ABC/XYZ classifications. - Challenge forecasts regularly:
Don’t take forecasts for granted. Question them. Do they agree with your gut feeling, with market feedback or with current developments? - Consistently integrate specialised knowledge:
Actively incorporate knowledge about market mechanisms, customer behaviour or product range strategies into the model setup and the interpretation of the results. Without context, even the best AI remains blind.
If you take these three points to heart, you will create the basis for making AI forecasts not only technically exciting, but also practically reliable.
And this is where we come full circle – time to summarise and look ahead.
Know your limits, utilise your potential – and learn more
Today, Mr Schneider has much more realistic expectations when it comes to AI forecasts. The initial euphoria has given way to a more reflected assessment – and this is precisely what has noticeably improved his planning quality. He uses AI where it really is better than the classic statistical methods and thus brings real added value. And he relies on his experience when the data is thin on the ground or the market is unstable. Instead of relying on one perfect solution, he relies on an intelligent combination: human judgement plus algorithmic analysis, statistics and AI where it fits best.
The conclusion: if you know the limits, you can utilise the strengths in a more targeted way. AI is not panacea – but it is a powerful tool if you use it correctly. It needs good data, a sustainable setup and people who know what they are doing.
📅 Are you curious? Then we cordially invite you to our online live session on 14 May at 10:00 am:
“Discover the potential of AI forecasts” (in German)
– Basics of AI forecasts
– Practical knowledge from real projects
– Case studies from planning
👉 Register here now, join the conversation and take the next step towards smarter forecasting!
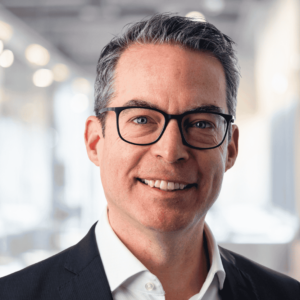